Pipeline
Publishing, Volume 5, Issue 8 |
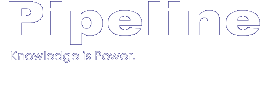 |
This
Month's Issue: |
What Now? The Future of Mobile Devices |
|
|
|
Integration, Master Data Management, and the Need for Migration
|
|
|
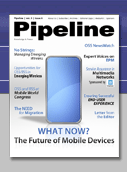 |
|
|

article page
| 1 | 2
| 3 |
difficult, time-consuming, and risky. Integration has offered an easier, cheaper, faster, and less intrusive method of achieving corporate goals – at least in the short term.
The drivers to achieve high-quality, complete, consistent and consolidated datasets are frequently the desire to improve processes and performance (stimulated, for example by a CRM, BI, or ERP initiative), the need to comply with new regulation (e.g. SOX), or the wish to implement service-oriented architectures (SOA) or software as a service (SaaS). Often, it’s only part-way through one of these initiatives that enterprises realize just how challenging their data issues are.
Recently, attention has focused particularly on the improvement of so-called master data, which comprises some of the most valuable data enterprises hold: information about people (customers, employees etc), assets, products, and places (office locations, geographic divisions, and so on). There is little new under heaven and earth, and so master data management (MDM) is an extension of previous concepts such as customer data integration (CDI) and product information management (PIM).
|
|
However, the truth is that master data management will not solve our data problems overnight, involves far more than just technology alone, and is not simply a matter of integrating data sets. |
|

Marketeers would have us believe that master data management technology can solve our data problems through the use of sophisticated technology. By plugging an MDM solution into existing applications, such as CRM, ERP, billing, inventory, and logistics, vendors argue that you can build a true, consistent view of data which can them be fed back into these systems. However, the truth is that master data management will not solve our data problems overnight, involves far more than just technology alone, and is not simply a matter of integrating data sets.
Integration is part of the answer, but it does not obviate the requirement to consolidate and migrate data. For example, it’s not uncommon for enterprises to have many
|
|
|
|

Managing master data is challenging for many large enterprises, and it is questionable whether simply integrating datasets is the best way forward. Take, for example, the situation that arises after a round of M&A activity. The enterprise acquires a complete set of master databases from the acquired company, each of which has dependent applications. The complexity combined with the requirement for day-to-day operations to continue undisrupted means that IT staff decide that they will leave the master databases physically separate but tie them together using a reconciliation process. Over time, however, and as the number of master databases and their dependent systems increases, the reconciliation process becomes more and more complex, unmanageable, and unreliable.
|
|

copies of customer data – this could be 20, 30, 40, or even 100 datasets. Customers are highly likely to be duplicated between these datasets leading to sub-optimal outcomes, such as a customer being mailed multiple times by the same company. To deliver the single, unified view of a company’s data that is desired, enterprises therefore need a data management strategy that fixes poor data management processes, improves data quality, and consolidates datasets,which will decrease both the risk of variant data as well as operational cost. The project should start small and grow over time, and the enterprise should begin by deciding its key priority – which might, for example, be to fix its customer data first. Each enterprise is
article page
| 1
| 2
| 3
| |
|
|
|
|
|
|
|